Customer Showcase: Half your deployment cost with minimum effort!
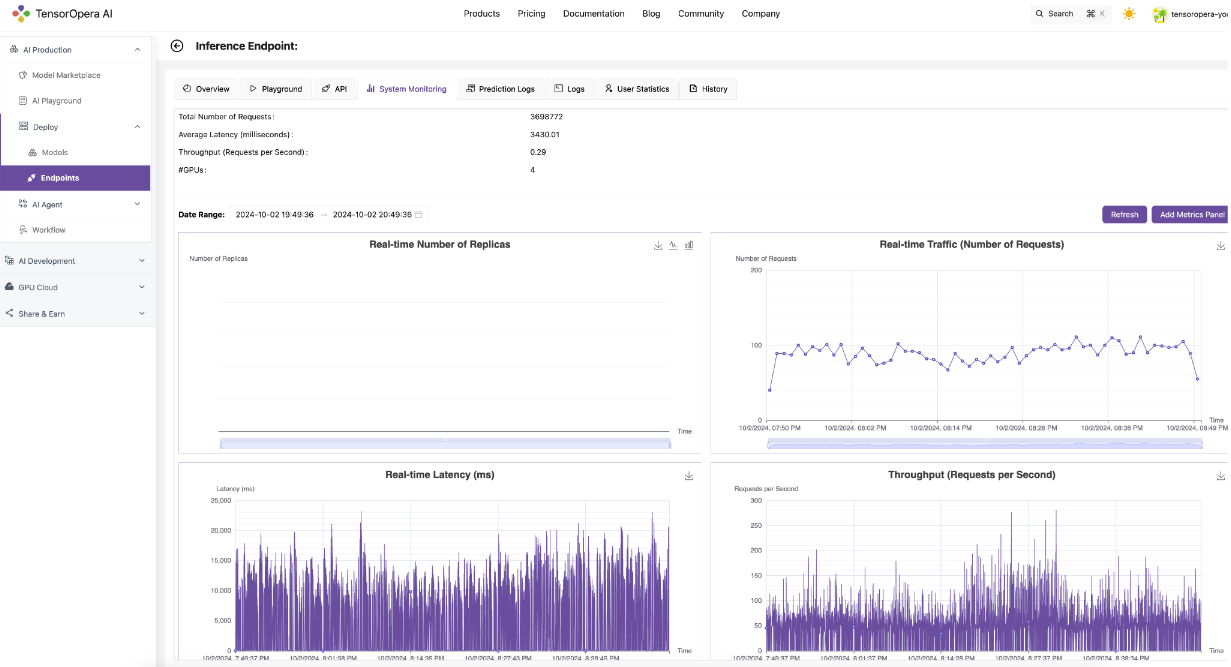
In a recent project, we want to showcase how a customer reduced their deployment cost by 50% by partnering with TensorOpera in less than a week!
In a recent project, a customer using a customized Llama3-70B model expressed a need for a high-performance, cost-effective solution for their AI model endpoint. They had previously fine-tuned their model on a different platform and communicated specific requirements for queries per second (QPS), machine type, and model/serving engine. Here's how TensorOpera successfully onboarded them, reduced costs, and increased efficiency.
Offer and Pricing
To meet the customer's needs, TensorOpera provided a compelling offer. We outperformed their previous provider, reducing the cost from $3.70 per hour on an H100 to our more affordable rate of $2.65 per hour. Reducing their bottom line by 33% with the same serving setup. Our smart autoscaling feature further optimized their GPU usage, minimizing the required dedicated GPUs while boosting overall performance even further.
Our approach involved initially shadowing their existing infrastructure to understand its intricacies. We then gradually optimized their setup, ensuring all adjustments aligned with their performance expectations while maximizing their cost-saving goals.
How We Onboarded the Customer
- Alignment on Requirements: After an initial customer meeting, we understood their current setup and agreed on a joint Slack channel for direct communication. Here, we collaborated closely with the customer to align on model specifications, serving engine, QPS targets, machine types, and quantities.
- Understanding Specific Demands: We delved into the customer’s unique needs, such as speculative decoding with Medusa and specific quantization methods, to tailor our solution precisely.
- Proof of Concept (PoC): We completed a quick two-day PoC to validate our approach and demonstrate the potential benefits.
- Shadow Testing and Optimization: Over the course of a week, we conducted a shadow test to gather feedback and refine the deployment. This allowed us to make adjustments and ensure that everything was set for full traffic migration.
- Traffic Switching: With everything optimized, we transitioned the customer’s traffic to the new setup seamlessly. Overall, reducing the customer's deployment cost by more than 50%!
- Dashboard Monitoring and Management: The customer now benefits from our comprehensive monitoring dashboard, which supports autoscaling and rolling updates for model iterations. They can also integrate their private API using the OpenAI standard format for seamless application integration.
Looking Ahead
As the customer’s traffic grows, TensorOpera can effortlessly add new GPUs to scale their infrastructure dynamically. Beyond the current text-to-text model, the customer has the flexibility to expand into text-to-image and voice cloning models, all readily available in TensorOpera's model hub.
We also provide a comprehensive model pipeline, allowing multiple models to work together, ensuring that the customer’s AI capabilities can grow in both scope and sophistication.
By partnering with TensorOpera, the customer has not only reduced their costs but also gained a scalable and efficient model deployment solution that can evolve with their needs.