FedML Celebrates One-Year Anniversary
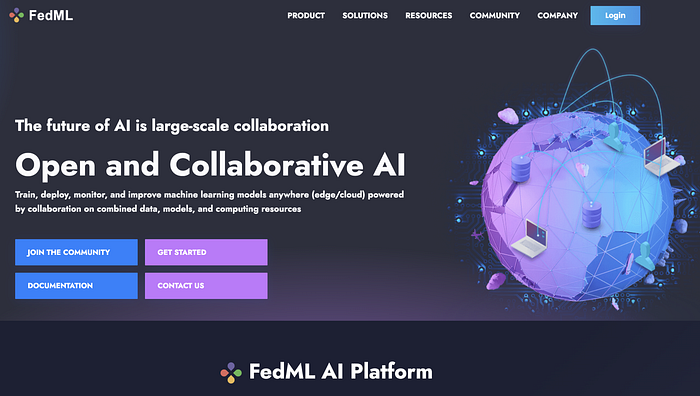
We are excited to announce that FedML has successfully reached its one-year milestone! Throughout this past year, we have upgraded our product portfolio going beyond federated learning, and enhanced it into a full stack of AI platforms with four interconnected MLOps: Community-Driven AI, FedML Train on the Edge, FedML Train on the Cloud, and FedML Serve Anywhere. We believe the future of AI is large-scale collaboration and shape our vision as “open and collaborative AI”.
Here’s a summary of the thrilling advancements at FedML over the past year.
1. Product — Upgrading towards a Full Stack MLOps
2. Traction of Community and Business Growth
3. Technology Achievement
4. FedML Team
1. Product — Upgrading towards a Full Stack MLOps
For its one-year anniversary, FedML has just released a completely revamped and redesigned AI platform and website.
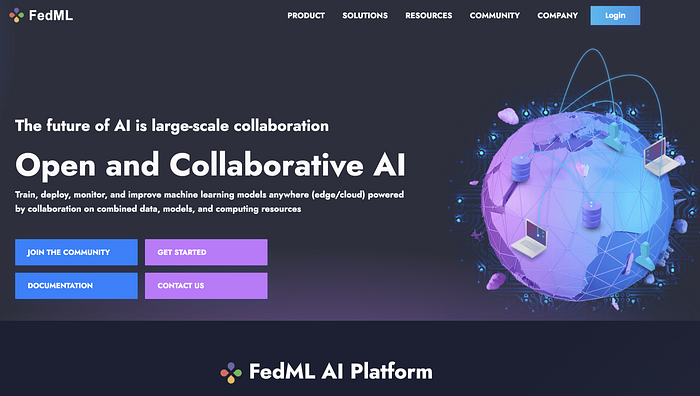
Website: https://fedml.ai
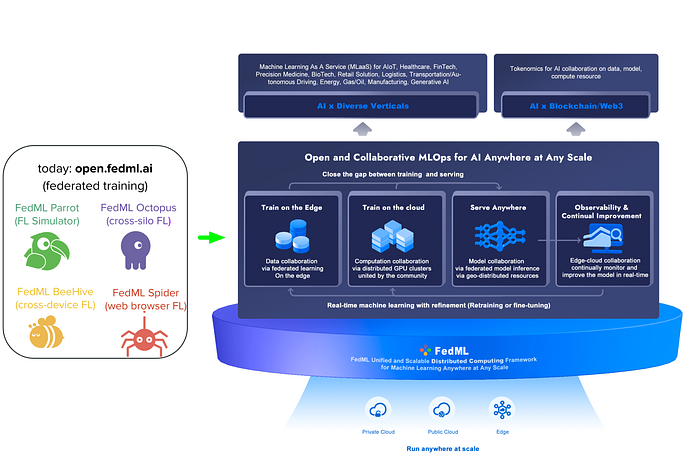
Platform: https://open.fedml.ai/
FedML AI ecosystem is now composed of four interconnected platforms:
(1) FedML Community-Driven AI (open source, application ecosystem, and AIxWeb3 platform)
(2) FedML Train on the Edge platform — Federated/decentralized/collaborative training platform across servers, mobile, IoT, web browsers
(3) FedML Train on the Cloud platform — Providing a decentralized cloud for collaborative training on combined compute resources
(4) FedML Serve Anywhere platform — Providing model-as-a-service over edge or the cloud for diverse AI verticals, as well as a platform for continual monitoring and improving AI models.
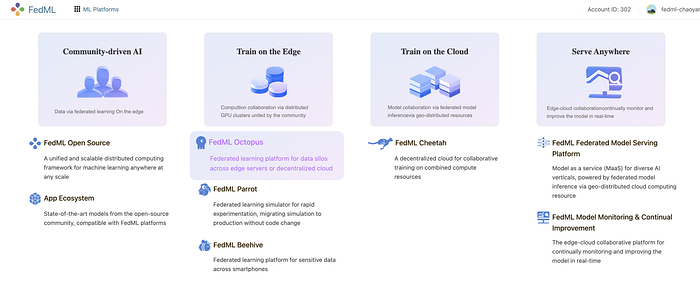
You can find FedML Octopus to have a tangible experience of how the upgraded platform looks like: https://www.fedml.ai/octopus
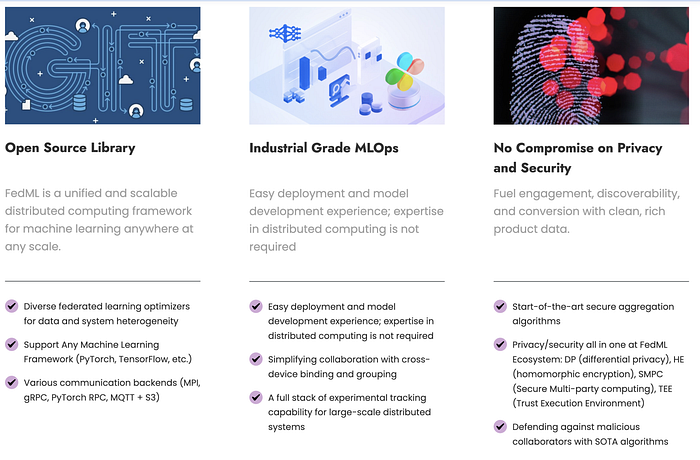
We’ve also enhanced features in aspects as follows.
Open Source Library
FedML is upgraded towards a unified and scalable distributed computing framework for machine learning anywhere at any scale.
- Support Diverse federated/collaborative/decentralized learning optimizers for data and system heterogeneity
- Support Any Machine Learning Framework (PyTorch, TensorFlow, etc.)
- Various communication backends (MPI, gRPC, PyTorch RPC, MQTT + S3)
Industrial Grade MLOps
Our MLOps is enhanced as follows:
- Easy deployment and model development experience; expertise in distributed computing is not required
- Simplifying collaboration with cross-device binding and grouping
- A full stack of experimental tracking capability for large-scale distributed systems
Please watch the demonstration at this link: https://www.fedml.ai/octopus
No Compromise on Privacy and Security
- Start-of-the-art secure aggregation algorithms
- Privacy/security all in one at FedML Ecosystem: DP (differential privacy), HE (homomorphic encryption), SMPC (Secure Multi-party computing), TEE (Trust Execution Environment)
- Defending against malicious collaborators with SOTA algorithms
Some examples can be found at: https://github.com/FedML-AI/FedML/tree/master/python/examples
2. Traction of Community and Business Growth
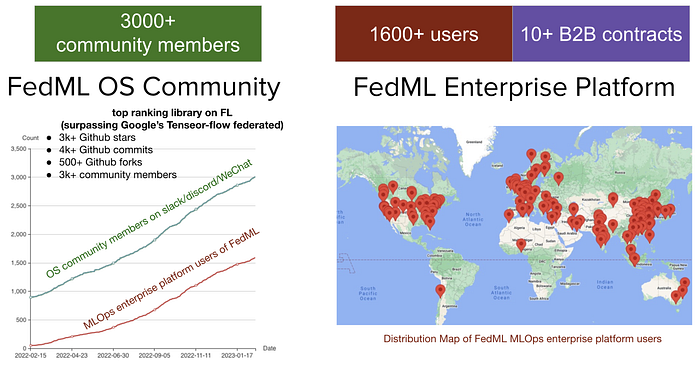
Traction on FedML Open Source Community
FedML open source community has 3000+ members on GitHub/Slack/Discord/WeChat worldwide. FedML surpassed TensorFlow Federated from Google, becoming the top-ranked open-source library on federated machine learning in the United States in November 2022.
Our open-source community also builds partnerships with well-known companies. Some representative examples are:
- Onboarding FedML to AWS ecosystem (https://aws.amazon.com/blogs/machine-learning/part-1-federated-learning-on-aws-with-fedml-health-analytics-without-sharing-sensitive-data/; https://aws.amazon.com/blogs/machine-learning/part-2-federated-learning-on-aws-with-fedml-health-analytics-without-sharing-sensitive-data/)
- FedML is connected to FATE/VMWare ecosystem
- Partnership with Owkin on releasing a Healthcare benchmark, presented at NeurIPS 2022 (https://owkin.com/publications-and-news/blogs/bridging-the-gap-between-federated-learning-theory-and-practice-with-real-world-healthcare-datasets)
- Partnership with Theta TV for web3 recommendation and advertisement.
Traction on FedML Enterprise AI Platform
FedML launched its MLOps in August 7th 2022. We’ve summarized the initial version in this blog: FedML AI platform releases the world’s federated learning open platform on the public cloud with an in-depth introduction of products and technologies! Here are some achievements of our FedML MLOps platform:
- In production ML, FedML MLOps platform has now reached more than 1700+ users worldwide (see the distribution map above), covering more than 500 universities and more than 100 small and medium-sized enterprises in North America, Asia-Pacific, Europe, and other regions around the world. The top 10 countries are from the United States, China, India, the United Kingdom, Korea, Germany, Singapore, Japan, and Canada.
- On FedML MLOps platform, AI/ML engineers/research scientists have deployed 3300 edge devices, and performed 6000+ training jobs.
- For enterprise business, FedML serves many AI verticals that need (1) a privacy-preserving and secure AI platform for learning from decentralized data; and (2) an edge-AI platform for training, deployment, monitoring, and continual improvement of their models at the edge. We have now reached 10+ enterprise B2B customers and a number of POCs spanning various AI verticals: Healthcare, Logistics, Retail, Smarty City/Home, generative AI, multi-cloud computing, and web3.
Traction on AI x Web3
What is particularly imaginative is probably “AI by the community for the community”. How will we change AI if the entire earth can collaboratively train generative AI, chatGPT-like LLM? We’ve summarized our vision at https://www.fedml.ai/web3. To shape this vision, we are excited to share with you our progress in both production and research.
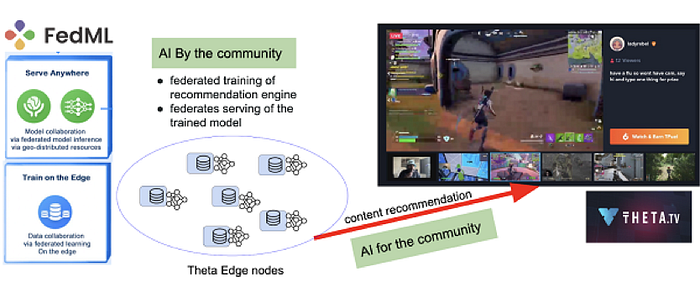
In an industrial platform, FedML announces partnership with Theta Network to empower Collaborative Machine Learning for Generative AI and Ad Recommendation. With the help of THETA’s 10000 nodes, FedML Collaborative AI infrastructure will have the opportunity to enhance quality and scalability significantly.
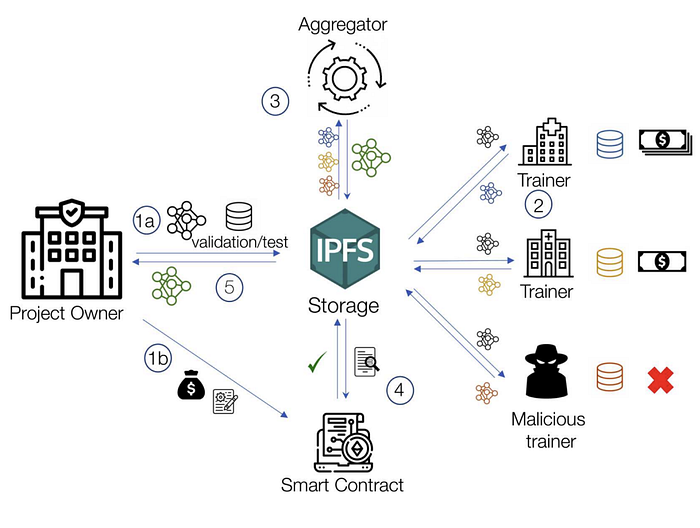
From the research side, we’ve released part of our work as a paper titled: “Proof-of-Contribution-Based Design for Collaborative Machine Learning on Blockchain” (https://arxiv.org/abs/2302.14031).
This paper studies three foundational problems in collaborative machine learning on blockchain:
(1) how to fairly and efficiently assess the contributions of each participant (even without “seeing” their contributing data)
(2) how to secure such systems against faulty or malicious participants (whether trainers or aggregators or contribution assessors)
(3) how to “prove” these heavy ML computations and bring them on the blockchain via scalable and efficient ZKML (i.e., zero-knowledge proof for various ML tasks, such as training, model aggregation, adversary detection, and contribution assessment).
This work would serve as the solid foundation of our AI x Web3 vision. We will release more progress in the future.
3. Technology Achievement
Research Publications
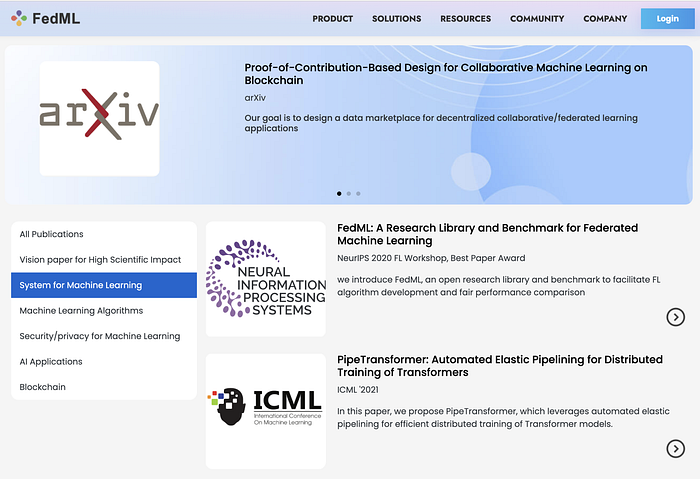
Until now, the FedML team has published over 50+ relevant publications in top AI and system venues. We’ve curated our publications at https://www.fedml.ai/researchPapers. The topics span machine learning algorithms, AI applications, blockchain, distributed systems, security, and privacy for machine learning.
Invited Talks and Panel List
FedML founders also delivered many keynotes about FedML technology at top-tier AI venues:
- Keynote speaker at the Workshop on “Cross-Community Federated Learning: Algorithms, Systems and Co-designs” (CrossFL-2022 ) at MLSYS 2022
- Keynote speaker at The 1st International Workshop on Federated Learning with Graph Data at ACM CIKM’22
- Keynote speaker at International Workshop on Trustworthy Federated Learning at IJCAI 2022 (FL-IJCAI’22)
- Keynote speaker at 2022 CVPR First International Workshop on Federated Learning for Computer Vision (FedVision)
- Keynote Speaker at 2022 Federated Learning for Natural Language Processing (FL4NLP) Workshop at EMNLP’22
- Keynote speaker 2022 Federated Learning for Private Web Search and Data Mining Workshop at WSDM’22
- MIT Invited Talk.
- MBZUAI invited talk: https://mbzuai.ac.ae/event/fedml-building-open-and-collaborative-machine-learning-anywhere-at-any-scale/
Workshop sponsorship
FedML Inc. has sponsored the following academic conferences/workshops
- FLSys @ MLSys 2023 — Workshop on Federated Learning Systems
- CrossFL 2022 @ MLSys 2022 — Workshop on Cross-Community Federated Learning: Algorithms, Systems, and Co-designs
- FL-IJCAI 2022 — International Workshop on Trustworthy Federated Learning
- FedGraph @ CIKM 2022 — Workshop on Federated Learning with Graph Data
- FL4NLP @ ACL 2022 — Workshop on Federated Learning for Natural Language Processing (FL4NLP)
More details can be found at https://www.fedml.ai/sponsorship
4. FedML Team
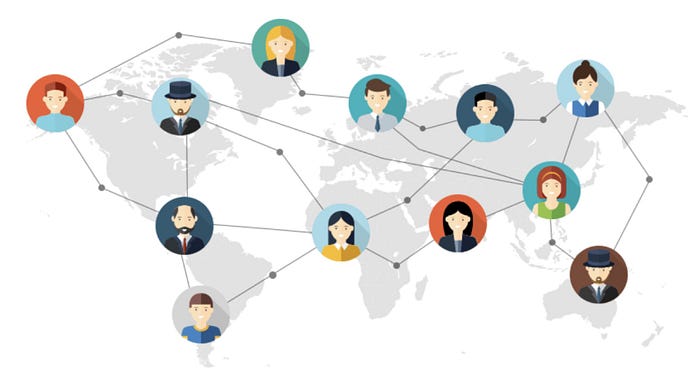
FedML was founded by Salman Avestimehr and Chaoyang He, and spun out from the University of Southern California at March 2022. Our team is distributed across the Bay Area, Los Angeles, and Hong Kong.
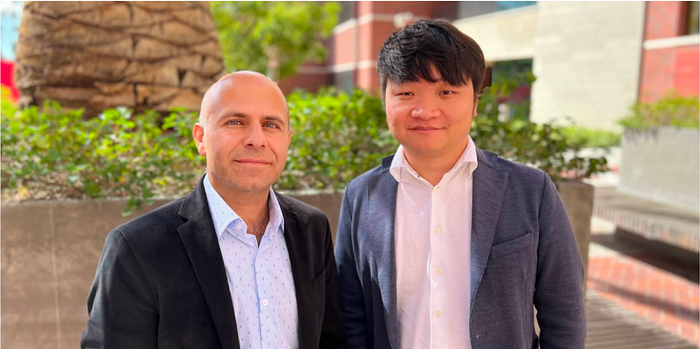
In addition to our work on AI and distributed systems, we have assembled a team to research AI tokenomics, which will help us bring our vision of “AI x Web3” to real-world products. The blockchain team includes top-tier blockchain engineers and professors from leading schools such as Stanford and UT Austin. We have also strengthened our advisory team with support from well-known figures.
Finally, we would like to express our gratitude to our community, partners, and investors for their support over the past year. We are excited to continue this journey together and work towards building a better future for open and collaborative AI.